Tensorflow 在默認設定下,每當執行一個程式,
他會先將看的到的 GPU 的 Memory 給全部佔滿,
所以就算只有用到一點點,其他程式也沒辦法搶到 Memory 資源去執行。
為了不要浪費資源,我們在執行程式前要先限制這個程式可以吃的 memory size
方法如下:
1 | import tensorflow as tf |
or
1 | config = tf.ConfigProto() |
可以使用以下指令來檢查GPU的使用率1
$ nvidia-smi
結果如下:
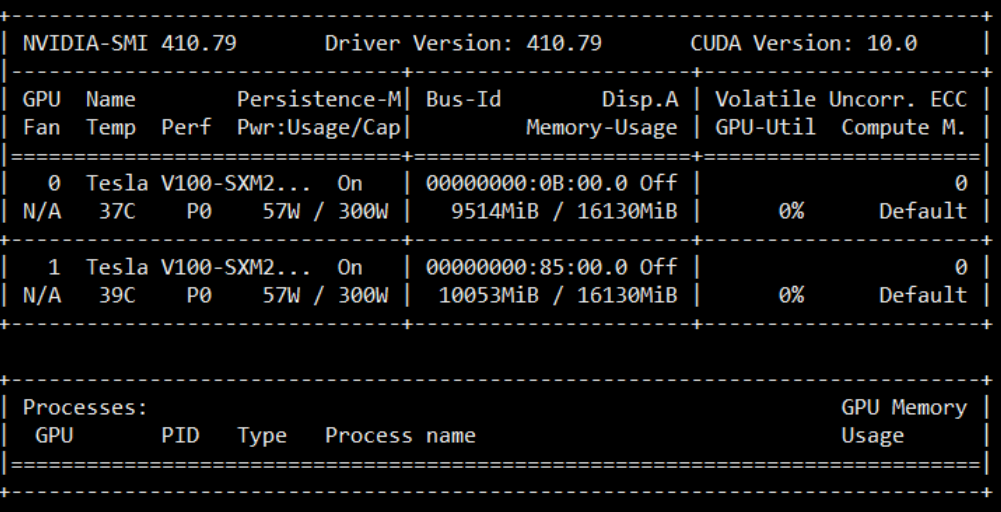
可以看到上面,就算限制了memory
但是他還是同時使用了兩個 GPU 去執行程式
如果我們想要只單純使用一個 GPU 去執行
在程式的”開頭”,我們必須加入設定
設定如下:
CUDA_VISIBLE_DEVICES 這個環境變數代表: 這個程式能看到的 GPU 有哪些
1 | import os |
而 GPU id 可以使用 $ nvidia-smi 來做查詢,或是使用下列語法來查詢 tensorflow 現在可以吃到的所有GPU狀態
1 | from tensorflow.python.client import device_lib |
結果如下:

Comments